Construction of risk prediction model of enteral feeding intolerance in severe neurosurgical patients based on machine learning algorithm
-
摘要:
目的 重症患者在应激情况下易发生胃肠运动功能障碍,其中喂养不耐受(FI)是常见临床表现,长时间FI会加重患者不良预后的风险。本研究旨在通过构建基于机器学习算法的成人神经外科重症患者肠内营养喂养不耐受风险预测模型,为临床提供有效的FI风险预测工具。 方法 选取2018年1月—2024年5月绍兴文理学院附属医院重症监护室收治的396例神经外科重症患者的临床资料并进行回顾性分析,分析神经外科重症患者发生FI的风险因素。将396例神经外科重症患者按照7∶3比例分成训练集(272例)和验证集(124例),采用3种机器学习算法在训练集中构建预测模型,使用验证集对模型进行评估。 结果 神经外科重症患者FI的发生率为35.86%(142/396)。影响因素分析表明,糖尿病病史、使用机械通气、使用血管活性药物、白蛋白水平<35 g/L、低血钾浓度是神经外科重症患者发生FI的独立危险因素。3种模型均具有良好的准确度和灵敏度,3种模型的Kappa值均>0.80,阴性和阳性预测值均>0.90,其中logistic回归分析模型的预测效能更高。 结论 3种模型的预测表现均较好,其中logistic回归分析模型的FI预测模型更具有优势,可有效识别神经外科重症患者的FI发生风险,有利于预测和识别FI高风险患者。 Abstract:Objective Severe patients are prone to gastrointestinal motor dysfunction when experiencing stress. Among the various clinical manifestations of this condition, feeding intolerance (FI) is a common occurrence. Prolonged FI increases the risk of a poor prognosis. The objective of this study is to develop a risk prediction tool for FI in adult neurosurgical patients with severe symptoms. The tool will be constructed using a machine learning algorithm to predict enteral nutrition feeding intolerance. The tool will help to reduce the incidence of FI and improve the prognosis of patients. Methods A retrospective analysis of the clinical data of 396 patients with severe neurological conditions in the intensive care unit of the Affiliated Hospital of Shaoxing University of Arts and Sciences from January 2018 to May 2024 was conducted. The study aimed to identify the risk factors for FI in neurosurgical critical patients Three machine learning algorithms were employed to construct an FI risk prediction model. The 396 neonatal intensive care unit (NICU) patients were randomly divided into a training set (n=272) and a validation set (n=124) according to a 7∶3 ratio. Machine learning algorithms were used to construct a prediction model in the training set, and the sensitivity and accuracy of the model were evaluated using the validation set. Results The incidence of FI in NICU patients was 35.86% (142/396). A subsequent analysis of the influencing factors revealed that a history of diabetes, the use of mechanical ventilation, the use of vasoactive drugs, an albumin level of < 35 g/L, and low blood potassium concentration were independent risk factors for FI in NICU patients. All three models demonstrated good accuracy and sensitivity. The Kappa values of all three models exceeded 0.80, and their negative and positive predictive values were greater than 0.90. The logistic regression analysis model exhibited superior predictive power. Conclusion The FI prediction performance of the three models is satisfactory, and the FI prediction model of the logistic regression analysis model has more advantages, which can effectively identify the risk of FI in neurosurgical critical patients. This is conducive for predicting and identifying patients with high risk of FI. -
表 1 FI发生风险的单因素分析
Table 1. Univariate analysis of FI risk factors
项目 不耐受组
(n=142)耐受组
(n=254)统计量 P值 性别[例(%)] 0.052a 0.820 男性 85(59.86) 155(61.02) 女性 57(40.14) 99(38.98) 年龄[例(%)] 0.013a 0.908 <60岁 78(54.93) 138(54.33) ≥60岁 64(45.07) 116(45.67) BMI(x±s) 23.76±2.26 23.82±2.17 0.260b 0.795 GCS评分(x±s,分) 4.37±0.85 5.64±0.93 13.435b <0.001 既往病史[例(%)] 高血压 75(52.82) 132(51.97) 0.026a 0.871 糖尿病 96(67.60) 88(34.65) 39.677a <0.001 恶性肿瘤 45(31.69) 81(31.89) 0.002a 0.967 治疗方法[例(%)] 手术 81(57.04) 146(57.48) 0.007a 0.933 机械通气 127(89.44) 155(61.02) 35.777a <0.001 放置脑室引流管 77(54.23) 153(60.24) 1.348a 0.246 抗生素 135(95.07) 191(75.20) 24.658a <0.001 血管活性药物 117(82.39) 157(61.81) 18.057a <0.001 镇痛剂 84(59.15) 153(60.24) 0.044a 0.834 实验室指标 血糖(x±s,mg/dL) 8.43±0.92 8.52±0.87 0.967b 0.334 血红蛋白(x,g/L) 113.43±13.57 112.59±15.28 0.546b 0.586 白蛋白<35 g/L[例(%)] 116(81.69) 132(51.97) 34.289a <0.001 血钾(x±s,mmol/L) 3.20±0.92 3.76±0.87 6.017b <0.001 血钠(x±s,mmol/L) 142.54±5.43 143.08±4.78 1.026b 0.306 C反应蛋白(x±s,mg/dL) 68.57±12.94 69.04±10.63 0.389b 0.697 营养液种类[例(%)] 0.121a 0.728 要素类 27(19.01) 52(20.47) 非要素类 115(80.99) 202(79.53) 营养液总量(x±s,mL) 1 325.64±21.05 1 248.65±23.41 32.521b <0.001 置管途径[例(%)] 0.165a 0.921 口胃管 4(2.82) 7(2.76) 鼻胃管 136(95.77) 242(95.28) 鼻肠管 2(1.41) 5(1.97) 注:a为x2值,b为t值。 表 2 FI发生危险因素的多因素logistic回归分析
Table 2. Multivariate logistic regression analysis of FI risk factors
变量 B SE Waldx2 P值 OR(95% CI) GCS评分<5分 1.909 1.241 2.366 0.124 6.741(1.469~12.526) 糖尿病病史 2.289 1.079 4.502 0.034 9.863(2.952~18.431) 实施机械通气 2.526 1.126 5.643 0.017 12.517(3.874~21.695) 使用抗生素 1.584 1.368 1.341 0.247 4.873(1.352~10.572) 使用血管活性药物 2.454 0.973 6.361 0.011 11.629(3.165~18.241) 白蛋白<35 g/L 2.724 1.104 6.088 0.014 15.236(4.728~25.863) 血钾 2.349 1.037 5.131 0.024 10.473(1.885~19.256) 营养液总量 2.109 1.447 2.124 0.145 8.237(1.529~15.694) 表 3 3种FI风险预测模型预测效能
Table 3. Prediction efficiency of three FI risk prediction models
模型 准确度 灵敏度 特异度 AUC Kappa值 阴性预
测值阳性预
测值Logistic 0.999 0.997 0.998 0.943 0.998 0.999 0.998 SVM 0.986 0.942 0.965 0.954 0.958 0.894 0.927 RF 0.974 0.953 0.948 0.879 0.873 0.958 0.968 表 4 3种FI风险预测模型混淆矩阵
Table 4. Confusion matrix of three FI risk prediction models
模型 实际值 预测值 不耐受=1 耐受=0 Logistic 不耐受组 47 0 耐受组 0 77 SVM 不耐受组 45 2 耐受组 3 74 RF 不耐受组 44 3 耐受组 2 75 注:表中数值为不耐受和耐受患者的例数。 -
[1] 赵玲玲. 住院危重患者早期肠内营养水平的相关因素研究[J]. 医药论坛杂志, 2020, 41(9): 128-130.ZHAO L L. Study on the related factors of early enteral nutrition level in critically ill hospitalized patients[J]. Journal of Medical Forum, 2020, 41(9): 128-130. [2] 陶维玲, 张红, 唐冬梅. 护士驱动下MDT管理模式在降低肠内营养患者喂养中断发生率中的应用[J]. 现代医药卫生, 2024, 40(14): 2422-2426. doi: 10.3969/j.issn.1009-5519.2024.14.020TAO W L, ZHANG H, TANG D M. Application of nurse-driven MDT management model in reducing the occurrence of feeding interruption in patients with enteral nutrition[J]. Journal of Modern Medicine & Health, 2024, 40(14): 2422-2426. doi: 10.3969/j.issn.1009-5519.2024.14.020 [3] 卜黎静, 程飞儿, 张爱琴, 等. 危重症患者肠内营养喂养不耐受风险预测模型的构建及验证[J]. 中华护理杂志, 2024, 59(15): 1877-1883.BU L J, CHENG F E, ZHANG A Q, et al. Development and validation of a prediction model for enteral feeding intolerance in critically ill patients[J]. Chinese Journal of Nursing, 2024, 59(15): 1877-1883. [4] 徐建英, 饶美霞. 微生态制剂的序贯性肠内营养对重型颅脑损伤患者营养状况的影响[J]. 浙江临床医学, 2022, 24(12): 1815-1817. doi: 10.3969/j.issn.1008-7664.2022.12.026XU J Y, RAO M X. Effect of sequential enteral nutrition with probiotics on nutritional status of patients with severe craniocerebral injury[J]. Zhejiang Clinical Medical Journal, 2022, 24(12): 1815-1817. doi: 10.3969/j.issn.1008-7664.2022.12.026 [5] 范小宁, 孙盼盼, 靳玉萍, 等. 护士主导的肠内营养喂养策略在神经重症患者中的应用[J]. 中华全科医学, 2022, 20(12): 2151-2155. doi: 10.16766/j.cnki.issn.1674-4152.002791FAN X N, SUN P P, JIN Y P, et al. Application of nurse-led enteral nutritional feeding strategies in neurological intensive care unit patients[J]. Chinese Journal of General Practice, 2022, 20(12): 2151-2155. doi: 10.16766/j.cnki.issn.1674-4152.002791 [6] SINGER P, ROBINSON E, RAPHAELI O. The future of artificial intelligence in clinical nutrition[J]. Curr Opin Clin Nutr Metab Care, 2024, 27(2): 200-206. doi: 10.1097/MCO.0000000000000977 [7] 四川大学华西循证护理中心, 中华护理学会护理管理专业委员会, 中华医学会神经外科学分会. 中国卒中肠内营养护理指南[J]. 中国循证医学杂志, 2021, 21(6): 628-641.Evidence-based Nursing Center, West China Hospital, Sichuan University; Nursing Management Professional Committee of Chinese Nursing Association; Chinese Neurosurgical Society, Chinese Medical Association. Nursing practice guideline for enteral nutrition in patients with stroke[J]. Chinese Journal of Evidence-based Medicine, 2021, 21(6): 628-641. [8] 钱传云. 2018ESPEN重症临床营养指南解读[J]. 中华重症医学电子杂志(网络版), 2019, 5(4): 384.QIAN C Y. Interpretation of 2018 ESPEN Clinical nutrition Guidelines for Severe diseases[J]. Chinese Journal of Critical Care & Intensive Care Medicine (Electronic Edition), 2019, 5(4): 384. [9] 丁佳莉, 刘晓光, 史甜, 等. 基于机器学习算法的重症脑出血患者肠内营养喂养不耐受风险预测模型构建[J]. 实用临床医药杂志, 2024, 28(12): 1-6. doi: 10.7619/jcmp.20240467DING J L, LIU X G, SHI T, et al. Construction of a risk prediction model for enteral nutrition feeding intolerance in patients with severe cerebral hemorrhage based on machine learning algorithms[J]. Journal of Clinical Medicine in Practice, 2024, 28(12): 1-6. doi: 10.7619/jcmp.20240467 [10] 李亚, 宋玉敏, 刘乐乐, 等. 基于前馈控制的早期肠内营养干预在重症患者中的应用[J]. 海南医学, 2023, 34(13): 1938-1941. doi: 10.3969/j.issn.1003-6350.2023.13.026LI Y, SONG Y M, LIU L L, et al. Application of early enteral nutrition intervention based on feedforward control in critically ill patients[J]. Hainan Medical Journal, 2023, 34(13): 1938-1941. doi: 10.3969/j.issn.1003-6350.2023.13.026 [11] 张丹. 间歇鼻饲不同泵注时间在重症监护治疗病房机械通气患者肠内营养中的临床效果[J]. 中国临床医生杂志, 2021, 49(7): 819-821. doi: 10.3969/j.issn.2095-8552.2021.07.019ZHANG D. Clinical effect of intermittent nasal feeding with different pumping time on enteral nutrition in patients with mechanical ventilation in intensive care unit[J]. Chinese Journal For Clinicians, 2021, 49(7): 819-821. doi: 10.3969/j.issn.2095-8552.2021.07.019 [12] SINGER P, ROBINSON E, RAPHAELI O. Gastrointestinal failure, big data and intensive care[J]. Curr Opin Clin Nutr Metab Care, 2023, 26(5): 476-481. doi: 10.1097/MCO.0000000000000961 [13] SONG J F, LI Z Y, YAO G J, et al. Framework for feature selection of predicting the diagnosis and prognosis of necrotizing enterocolitis[J]. PLoS One, 2022, 17(8): e0273383. DOI: 10.1371/journal.pone.0273383. [14] 吕玉颖, 曹志新, 钟晖, 等. 早期肠内营养治疗对重症呼吸衰竭患者营养状况及肺功能的影响[J]. 中国食物与营养, 2024, 30(5): 84-88. doi: 10.3969/j.issn.1006-9577.2024.05.014LYU Y Y, CAO Z X, ZHONG H, et al. Effect of early enteral nutrition therapy on nutritional status and lung function in patients with severe respiratory failure[J]. Food and Nutrition in China, 2024, 30(5): 84-88. doi: 10.3969/j.issn.1006-9577.2024.05.014 [15] 陈翻享, 莫绮君, 方笑媚. 通降胃气法干预结合床旁超声监测对机械通气重症患者早期肠内营养喂养不耐受的影响[J]. 现代消化及介入诊疗, 2024, 29(5): 578-582.CHEN F X, MO Q J, FANG X M. Effect of regulating gastric qi combined with bedside ultrasound monitoring on early enteral nutritional feeding intolerance in patients with severe mechanical ventilation[J]. Modern Interventional Diagnosis and Treatment in Gastroenterology, 2024, 29(5): 578-582. [16] 周甜, 李贞, 王猛霞, 等. 信息化肠内营养耐受性动态管理干预在急诊重症监护室患者中的应用效果[J]. 中国社区医师, 2024, 40(13): 111-113.ZHOU T, LI Z, WANG M X, et al. Application Effect of Information-Based Dynamic Management Intervention for Enteral Nutrition Tolerance in Emergency Intensive Care Unit Patients[J]. Chinese Community Doctors, 2024, 40(13): 111-113. [17] 杨茂凡, 周会兰, 陈柯宇, 等. ICU患者肠内营养喂养不耐受风险预测模型的系统评价[J]. 护士进修杂志, 2024, 39(14): 1512-1517.YANG M F, ZHOU H L, CHEN K Y, et al. Systematicevaluation of risk prediction models for enteral feeding intolerance in ICU patients[J]. Journal of Nurses Training, 2024, 39(14): 1512-1517. [18] 赵海霞, 肖婷, 胡敏, 等. 基于肠内营养耐受性评估表的早期肠内营养支持对重症监护病房患者疾病治疗的价值研究[J]. 陕西医学杂志, 2024, 53(5): 637-640. doi: 10.3969/j.issn.1000-7377.2024.05.013ZHAO H X, XIAO T, HU M, et al. Value of early enteral nutrition support therapy for patients in intensive care unit based on enteral nutrition tolerance assessment sheet[J]. Shaanxi Medical Journal, 2024, 53(5): 637-640. doi: 10.3969/j.issn.1000-7377.2024.05.013 [19] 李红玲, 王昌成, 葛晓璐, 等. 重症胰腺炎患者早期肠内营养治疗前后肠黏膜屏障的变化及喂养不耐受危险因素分析[J]. 临床和实验医学杂志, 2024, 23(8): 809-812. doi: 10.3969/j.issn.1671-4695.2024.08.007LI H L, WANG C C, GE X L, et al. Changes of intestinal mucosal barrier and risk factors of feeding intolerance in patients with severe pancreatitis before and after eraly enteral nutrition treatment[J]. Journal of Clinical and Experimental Medicine, 2024, 23(8): 809-812. doi: 10.3969/j.issn.1671-4695.2024.08.007 [20] 陈小颉, 段霞, 郑微艳, 等. 肠内营养病人喂养不耐受风险预测模型的系统评价[J]. 肠外与肠内营养, 2024, 31(2): 107-113.CHEN X J, DUAN X, ZHENG W Y, et al. A systematic review of risk prediction models for feeding intolerance in patients receiving enteral nutrition[J]. Parenteral & Enteral Nutrition, 2024, 31(2): 107-113. [21] LONGATO E, ACCIAROLI G, FACCHINETTI A, et al. Simple linear support vector machine classifier can distinguish impaired glucose tolerance versus type 2 diabetes using a reduced set of CGM-based glycemic variability indices[J]. J Diabetes Sci Technol, 2020, 14(2): 297-302. doi: 10.1177/1932296819838856 -
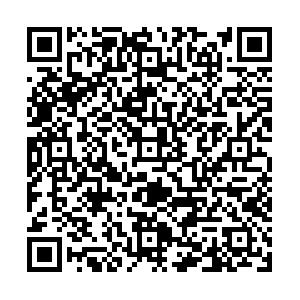
计量
- 文章访问数: 5
- HTML全文浏览量: 3
- PDF下载量: 1
- 被引次数: 0